Six Steps to Generate Quick Wins with Your Data and Analytics Projects
- Digital Hub Team
- Dec 15, 2020
- 4 min read
Generating quick wins with data and analytics is key to engaging the stakeholders and kick starting the learning journey that every organization needs to accelerate their recovery post COVID.
However, odds are against anyone who's after quick wins in this space. Gartner predicts that "Through 2022, only 20% of analytic insights will deliver business outcomes." This is alarming for leaders who are responsible for data and analytics and delivering business value.
A proven strategy to counter these odds is to build a portfolio of high impact use cases and launch several experiments in parallel. However, this will increase the project execution cost due to demand on infrastructure and resources required to enable the use case. But there is a solution to this challenge.
The question therefore is how to generate quick wins using a portfolio approach while keeping the cost down? Below is a tested and proven approach by Integra Data Science team that would help you identify, validate, research and deploy your quick wins using an on-demand model that will keep the cost down.
Identify your organizations strategic objective
Most organizations have well-established strategic objectives. As an example, reducing cost is a key objective in Oil and Gas industry in Canada that can be achieved through increasing productivity, eliminating the cost of unplanned maintenance and downtimes, and increasing outputs.
Now that you have figured out the strategic objective, you need to go to the next step and identify the use cases that fit into this objective.

Define the relevant use cases that meet the corporate strategic objective
This is a very critical step in making analytic projects successful. While exploring improvement opportunities in your organization, you may come across many analytic applications. Again, let's draw from our experience in Oil and Gas industry and hopefully you can identify parallels in your industry. Oil and Gas is an asset intensive industry, therefore maintenance and reliability cost are typically high. So in order to reduce cost (strategic objective), you can look at predictive maintenance application for pumps, pressure valves, compressors, and storage tanks, or failures in pipelines and identify many improvement opportunity.
Keep in mind that the scope you choose should be small enough that you are able to perform your end-to-end analysis within 8-12 weeks.
Onboard the subject matter experts
It is critical for the subject matter expert to believe in value of a data science project. Their opinions and direction to the team will break or make your project. They must also have time to provide meaningful contribution throughout the process and be vested in the end goal. Make sure their leader is also onboard to ensure additional support. Ultimately they are the stakeholders that should support your project's insights and help turning them into actions. If you have already established the Analytics Centre of Excellence (COE), you are off to a great start.
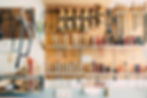
Choose your toolkit
Tools and technologies should act as enablers and accelerators and help you to succeed in your data and analytics efforts, however not having access to the right toolkit and integration complexities can act as a barrier and slow down your data science projects progress. You will need tools to wrangle and organize your date, build models, generate visualizations and potentially integrate back to existing systems. For the discovery phase of your project you might already have the necessary tools installed on your laptop but if you are doing this in a large organization you would need to learn about what tools and process have been established by your organization's Information Service. Plus using a laptop will limit you to your laptop's processing power and limit your organization's ability to access the insight you have generated. This is where things can get complicated with your corporate policy or cost can pile up as you put demand on other parts of the organization from infrastructure and resource prospective.
This is where we used our platform to deliver several parallel projects while maintaining cost. The on-demand feature of digitalhub.io means you can have several projects at the same time but only accumulate any significant cost for those that are actively being used. Your team can start, stop and delete the project infrastructure on-demand.
If you have a small or midsize organization, we highly recommend checking out our platform Digital Hubâ„¢ for its ease of use, collaboration and convenience features. The Platform will provide the necessary tools for data wrangling, data modelling, visualization and collaboration in a cost-effective and convenient package.
Gather existing data
At this stage, you're still validating the use case so go for small data not Big Data. Spend time to review any relevant business process documentations that explains how things work and what data is generated. This helps you understand the anomalies in the data and determine what adjacent data sources (upstream and downstream processes) might be useful in future.
Validate the initial hypothesis
It is very common to learn through the first few steps and adjust your hypothesis. For example, you might have made assumptions that are no longer true, or you might be missing data necessary to back your use cases. Don't be afraid of failing early and re-setting your project; That's why agile methodologies work well in this case. Failing for these reasons is meaningful and adds to your organization's learning capacity. If your initial hypothesis is still valid, you're ready to start planning and development of your data science project.
Good luck!
Organizations must take a portfolio approach to delivering successful data science initiatives. Embrace the fail fast culture and reward those individuals who are innovating with data.
Babak Shafiei, CEO & Founder Integra Data and Analytic Solutions